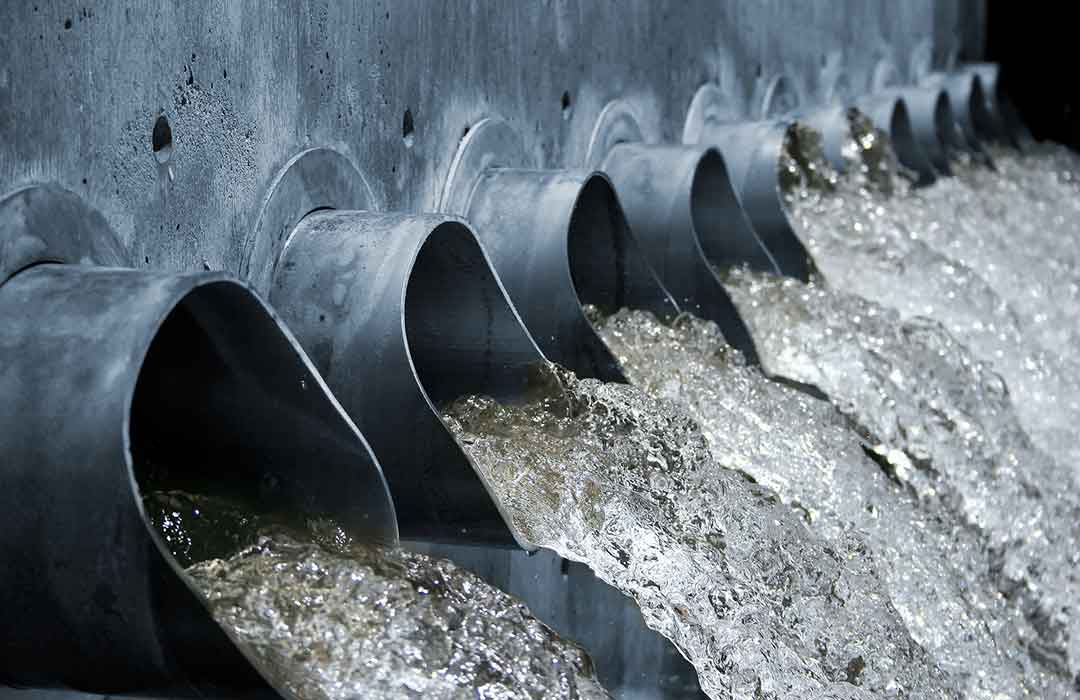
Community Water System Risk & Resiliency Assessment
March 27, 2020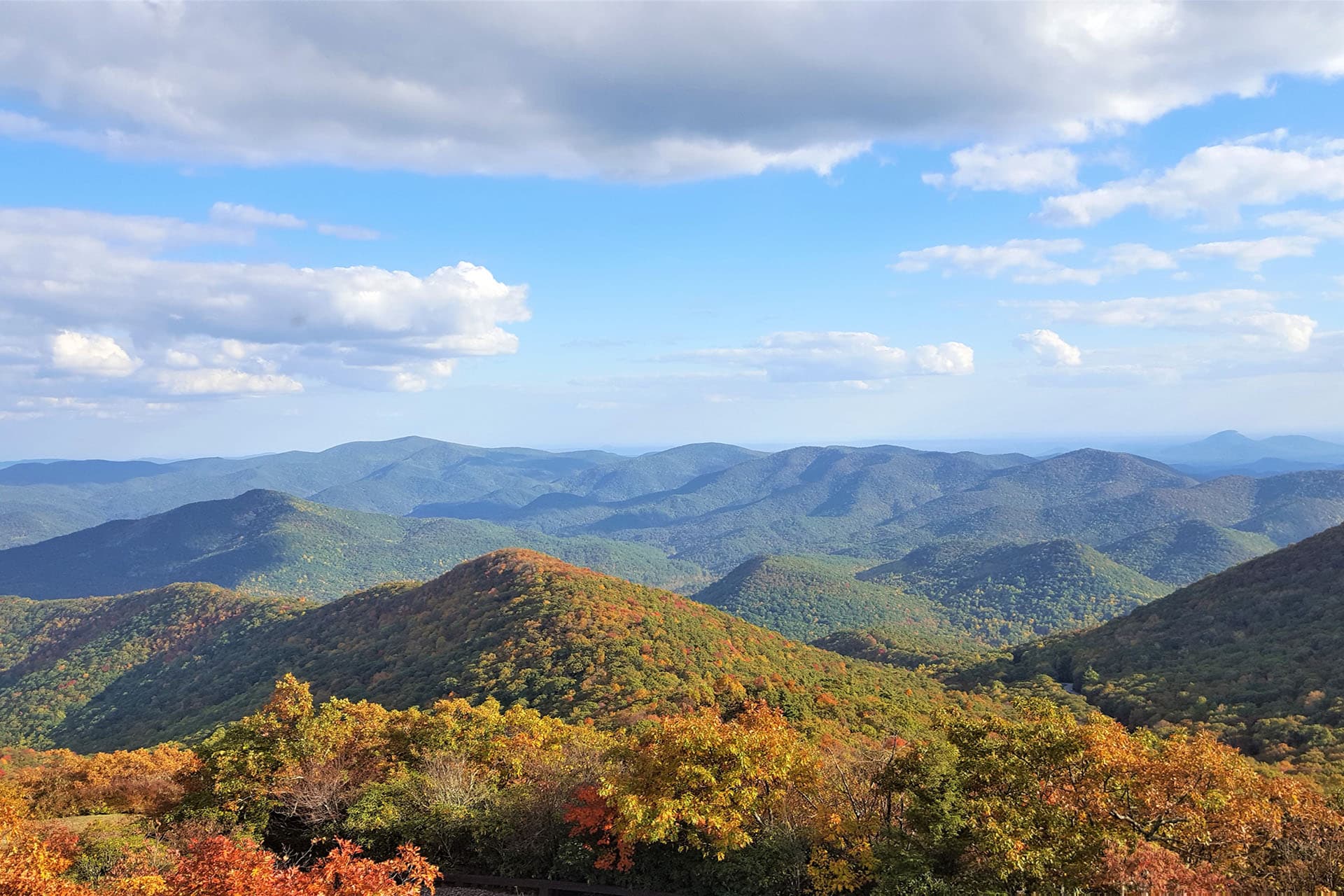
Regional Economic Disaster Recovery
March 20, 2020
SITUATION
SPEAR is a DOD web-based program that provides the capability to aggregate, display, and analyze human readiness data at various echelons of detail, delivering a clear picture of current readiness to the user. iParametrics is providing external machine learning modeling to support predictive and prescriptive outcomes within SPEAR and the DODs Human Performance Program.
TASK
Our task was to utilize different machine learning and analytics techniques to provide predictive and prescriptive outcomes utilizing military healthcare data.
ACTION
We developed a custom unsupervised learning clustering model to identify the military performance indicators (PI) that standout uniquely amongst the military branches by performance and intervention (amongst over 300+ PIs). Clustering and pruning algorithms sectioned out the feature outliers (both large and small) and element-wise values. Ranges of PI feature and value pairs were left over that become the primary pool for further selection. Component analysis algorithms helped identify the top PIs that explained the majority of variance for soldier and sailor performance. In addition, we developed a graphical user interface that allows the user to visualize various metrics related to human performance improvement and interventions and then to explore various “what if” scenarios by manipulating various filters and controls.
RESULT
In its current form, the iParametrics performance interface utilizes a graphical user interface that allows the user to visualize various metrics related to human performance improvement and interventions and then to explore various “what if” scenarios by manipulating various filters and controls. In order to maximize the effectiveness of the interface, iParametrics applied machine learning approaches to select optimal performance indicators. In other words, in addition to utilizing advanced data analysis techniques, iParametrics utilized “feature engineering” techniques to determine which performance indicators (out of several hundred) were most highly correlated with positive outcomes. The machine learning algorithms and the user interface allows users to dynamically compare different populations across multiple dimensions as a means of determining optimal combinations of factors to optimize soldier health and human performance.
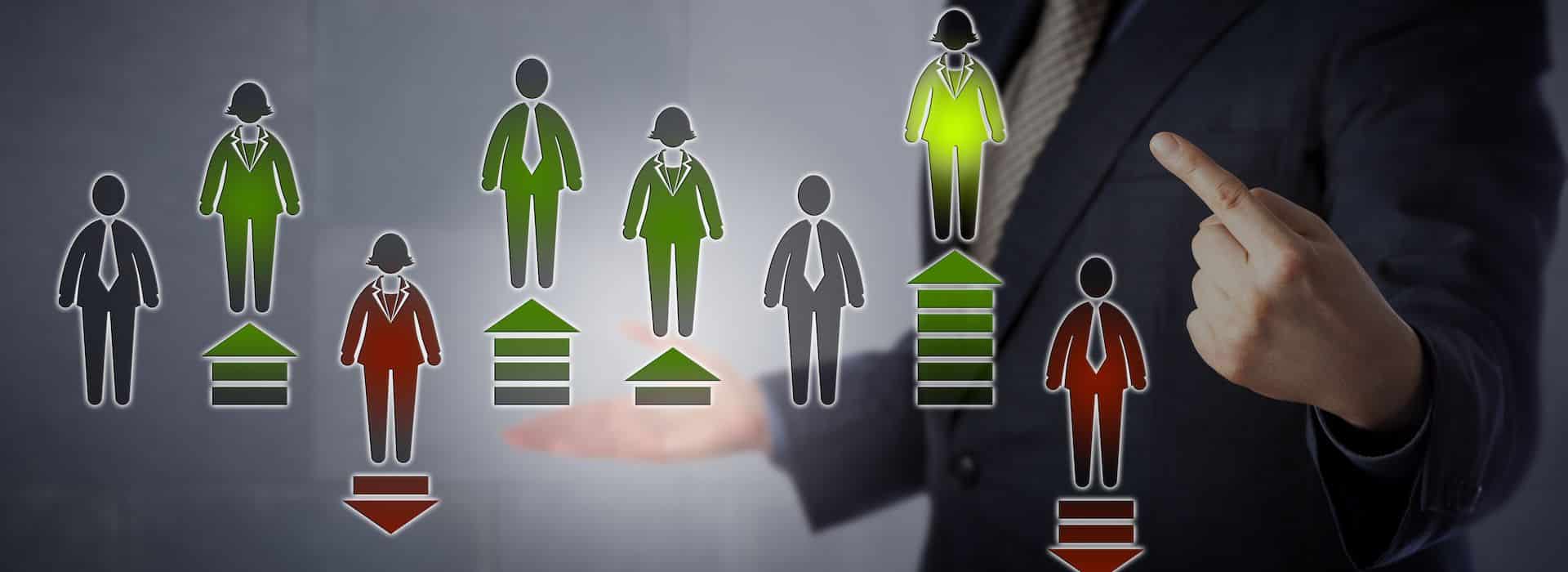